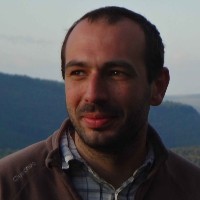
The talk will be in COL.1.06.
Title: Computation-information gap in high-dimensional clustering.
Abstract: We investigate the existence of a fundamental computation-information gap for the problem ofclustering a mixture of isotropic Gaussian in the high-dimensional regime, where the ambientdimension p is larger than the number n of points. The existence of a computation-information gap ina specific Bayesian high-dimensional asymptotic regime has been conjectured by Lesieur et al. (2016)based on the replica heuristic from statistical physics. We provide evidence of the existence of such agap generically in the high-dimensional regime p >n, by (i) proving a non-asymptotic low-degreepolynomials computational barrier for clustering in high-dimension, matching the performance of thebest known polynomial time algorithms, and by (ii) establishing that the information barrier forclustering is smaller than the computational barrier, when the number K of clusters is large enough.These results are in contrast with the (moderately) low-dimensional regime n> poly(p,K) where there isno computation-information gap for clustering a mixture of isotropic Gaussian. This is based on a jointwork with Bertrand Even and Christophe Giraud (Paris-Saclay).
Biography: Website